How Data-Driven Linear helps advertisers optimize their media strategy
EDO’s Scott Grunther discusses the evolution of DDL and the benefits of behavioral outcomes for advertisers
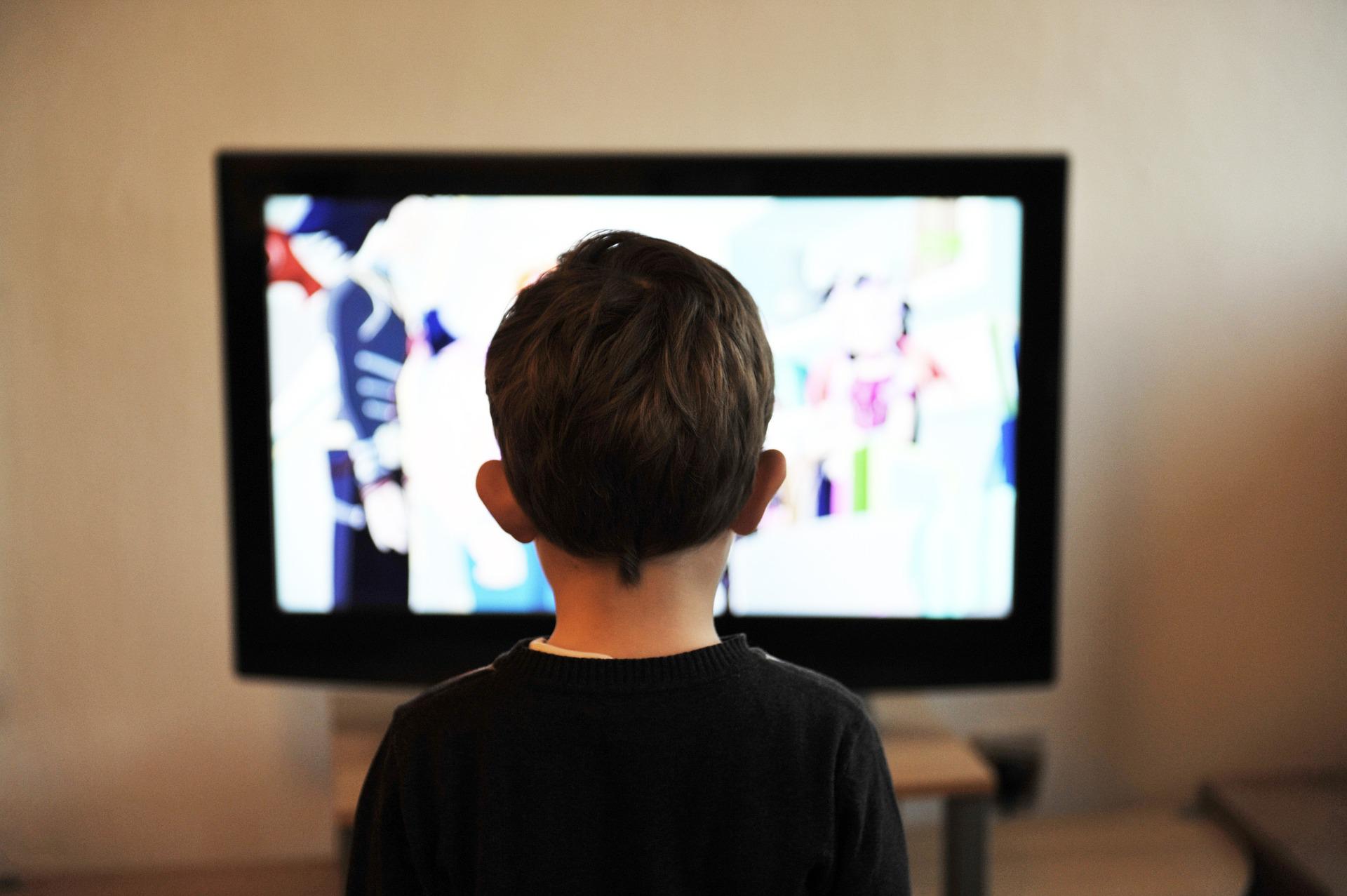
Sponsored Content from EDO
Brands and advertisers have grown accustomed to using impressions to measure their campaigns, but unfortunately, all impressions are not measured equally and can provide a skewed view of a campaign’s effectiveness. Enter “Data-Driven Linear.” The concept has evolved and can be leveraged beyond simply planning against an audience segment to optimize a campaign using behavioral outcomes. This approach delivers metrics that account for the premium content environment and ensures advertisers are planning, optimizing and measuring TV campaigns using the same data.
In this Q&A, Scott Grunther, head of media partnerships at EDO, a data and analytics company that measures predictive outcomes for Convergent TV, discusses what "Data-Driven Linear" really means, how to leverage DDL beyond simply planning against an audience segment and how behavioral outcomes are an ideal measure to optimize and plan media buys in near real-time.
How do you define "Data-Driven Linear"? Why is it a more precise approach for marketers to reach their target audiences?
Grunther: Data-Driven Linear was initially established by networks and agencies as a way to go beyond traditional age and gender demographics. The idea behind it: use technology, automation and data to target TV viewers based on audience concentration and increase the precision of a media campaign. If you are an insurance brand, are you looking for the highest rated show on P25-54, or the best programs to reach people in the market for insurance.
Longstanding data sets such as MRI Fusion join Nielsen ratings and attitudinal surveys to identify environments that reach the highest concentration of a particular audience segment. However, we have increasingly found that it’s not enough to solely target certain audiences and plan based on audience concentration. While advertisers typically stop at setting media plans based on high-level audience information, they are missing a crucial element that will help them optimize their media strategy: behaviors and outcomes data – and actual engagement with a program and the commercials within it.
For EDO, Data-Driven Linear means taking the typical media planning process one step further by leveraging traditional audience metrics with the strength of behavioral outcomes to optimize plans. Advertisers can – and should – incorporate the engagement levels of a particular programming environment, such as program genre, daypart or a specific show, to ensure they are reaching the right audience in the right environment.
Data-Driven Linear empowers brands and networks to take their media planning and optimization to another level. With DDL, they can harness the underlying power of the environment that drives users to engage with a brand, making it a more effective approach than what they have been historically accustomed to.
Why should media companies look beyond impression measurements? What role does the programming environment play in determining a brand’s ability to drive consumer engagement and behavioral response?
Grunther: Agencies and TV advertisers want to know more than just how many viewers saw an ad – they want to know if their TV investment is driving actual consumer responses - in near real-time.
While impressions are a natural starting place for measurement, not all impressions – and marketing signals, more generally – are created equal. Traditionally, marketing signals such as impressions, reach or sentiment don’t say what’s really working on TV. Take, for example, survey-based methodologies. They are often served far past the campaign’s end date, making it difficult for advertisers to understand what lessons to apply to in-flight and future campaigns.
For example, certain programs, genres or dayparts may perform better in driving high levels of engagement with ads, with engagement levels above what the impression count would suggest. In order to plan and optimize TV campaigns based on engagement, marketers require a robust historical and behavioral dataset that is stable and predictive.
We find that the added step creates more of an impact for the campaign because advertisers aren’t just reaching an audience based on impressions or how many TV viewers were exposed to an ad. The evolution of Data-Driven Linear allows advertisers to reach an audience that is more inclined to purchase the product or engage with the brand than previous methods of targeting.
When you target based on traditional demos, you assume that the people who fall within certain buckets all think and behave similarly – but really, they don’t necessarily want to purchase the same products. Advanced advertising methods help narrow in on the unique attitudes and behaviors of viewers. This ensures marketers have the highest likelihood of driving actionable KPIs, and most importantly, engaging viewers.
What’s the biggest challenge DDL is solving for marketers?
Grunther: The biggest challenge with Data-Driven Linear is when advertisers plan and measure campaigns based on different datasets. Often, networks and advertisers will use one dataset to measure the performance of a DDL campaign, but they have not factored it as an input into creating or optimizing the media plan. This is a mistake.
The core issue here is that if you plan a campaign using audience segmentation data, and then measure performance using, say, behavioral outcome data, then you may reach a high concentration of a particular audience, but in a show or daypart that is just not necessarily the most engaging for the brand or category. The opportunity for marketers and networks lies in planning and optimizing off the same datasets to increase the likelihood of campaign success compared to relative benchmarks. Ideally, marketers want to reach the right audience in the right environment, thus maximizing the chance of a strong performing campaign.
In addition, behavioral outcomes are an ideal measure to optimize and plan media buys, as they enable real-time data to empower in-flight media optimization. While advertisers have become accustomed to the “set it and forget it” mentality, outcomes enable them to make adjustments in-flight based on performance as the campaign is running. In fact, a huge benefit of using behavioral outcomes is that it empowers both buyers and network sales teams to measure TV just as they would a digital platform, which historically hasn’t been possible.
This is particularly important for CMOs, who feel the pressure from their internal C-suite colleagues, especially the chief financial officer, to measure all their channels and tactics against a financial outcome, such as how marketing and media drive incremental revenue, or how a TV ad drives a consumer to purchase.
How can embracing an “outcomes approach” to measurement better illustrate the effectiveness of TV ad campaigns and high-value media placements?
Grunther: Data-driven linear creates for more efficient media TV buys and enables better cross-platform planning and optimization. Without consumer behavioral measurement, advertisers lose the contextual nuance of the content. As measurement evolves, metrics will need to account for the premium content environment.
When advertisers use historical, attitudinal audience segmentation, it’s typically based on self-reported survey data. With predictive behavioral outcome measurement, TV doesn’t need pixels or tags to know what’s working. This data provides a unique opportunity for buyers and sellers who have the desire to move further down the funnel.
Last year, for example, Univision announced a partnership with EDO, Mediahub and datafuelX to demonstrate the potential for true data-driven media planning for marketers. Univision now leverages proprietary consumer behavior data to predict engagement performance for advertiser campaigns across its linear networks, using behavioral measurement and analytics from EDO as a currency to show which programming environments will be strongest for viewer engagement with the brand being advertised.
Data-driven investment is about more than targeting an audience, it’s about buying more effective impressions. In a world of massive media fragmentation and endless consumer choice, leveraging proven TV metrics and behavioral currencies is critical to investing in higher quality TV plans and placements.
What do you think the 3 big trends will be in DDL next year?
Grunther: I see three core DDL trends in 2023:
- Further seamless integration between audience segmentation data and behavioral outcome data;
- Buyers and sellers will increasingly plan, optimize, measure and guarantee against behavioral outcomes; and
- Buyers and sellers will demand faster, real-time data for use in DDL than is available with just survey-based approaches.
About Scott Grunther: Scott is head of media partnerships for EDO, leading commercial activity and overseeing EDO's business with the Ad Sales, Research, Marketing and Advanced Advertising teams at TV Networks and Publishers. Scott has worked in the advertising and media industries for his entire career, with the past 16 years spent building businesses and leading teams within the marketing research, data and insights arena. His area of focus and expertise has been on delivering advertising effectiveness insights and intelligence to both media companies and marketers. Scott began his career in television ad sales at CNN, and has also worked for a number of startup companies in the advertising and data technology space.
About EDO
EDO was co-founded in 2015 by award-winning actor, filmmaker, and entrepreneur Edward Norton and PhD economist, poet, and entrepreneur Daniel Nadler.
EDO's headquarters are in three exciting locations: Culver City in Greater Los Angeles, the SoHo district of New York City, and SOMA in San Francisco. These three places are the springboard from which EDO’s team supports clients nationwide as they strive to generate greater value from their creative efforts and media investments.
NEXT TV NEWSLETTER
The smarter way to stay on top of the streaming and OTT industry. Sign up below.